本文共 1433 字,大约阅读时间需要 4 分钟。
1 注意/在清单文件中配置activity/theme=
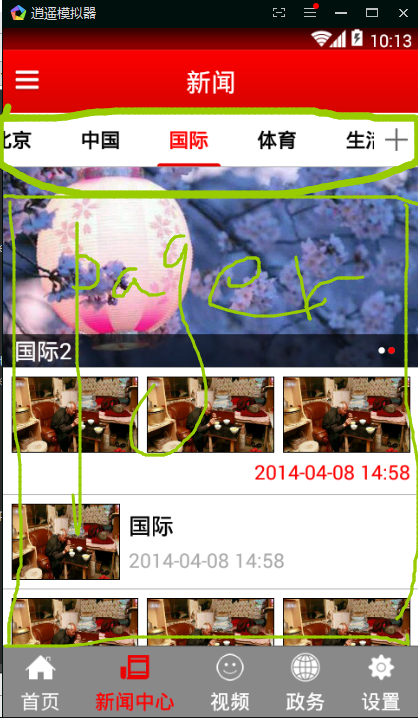
android:theme="@style/Theme.PageIndicatorDefaults">
//
2 (adpter中添加getPageTitle)
//1.给viewPager设置数据适配器 MyPagerAdapter myPagerAdapter = new MyPagerAdapter(); pager.setAdapter(myPagerAdapter); //2.指示器和viewPager进行绑定 indicator.setViewPager(pager);
3 重点(修改选中文字的颜色,背景)
values/style/tabPageIndicator
@android:color/transparent 为加背景,,不能用@null
<item name="vpiTabPageIndicatorStyle">@style/Widget.TabPageIndicator</item>//这里
</style>
<style name="Widget"> </style> <style name="Widget.TabPageIndicator" parent="Widget"> <item name="android:gravity">center</item>
<item name="android:background">@drawable/vpi__tab_indicator</item>//背景
<item name="android:paddingLeft">22dip</item> <item name="android:paddingRight">22dip</item> <item name="android:paddingTop">12dp</item> <item name="android:paddingBottom">12dp</item> <item name="android:textAppearance">@style/TextAppearance.TabPageIndicator</item> <item name="android:textSize">12sp</item> <item name="android:maxLines">1</item> </style> <style name="TextAppearance.TabPageIndicator" parent="Widget"> <item name="android:textStyle">bold</item>
<item name="android:textColor">@color/vpi__dark_theme</item>//文字颜色
</style> <style name="Widget.IconPageIndicator" parent="Widget"> <item name="android:layout_marginLeft">6dp</item> <item name="android:layout_marginRight">6dp</item> </style></resources>